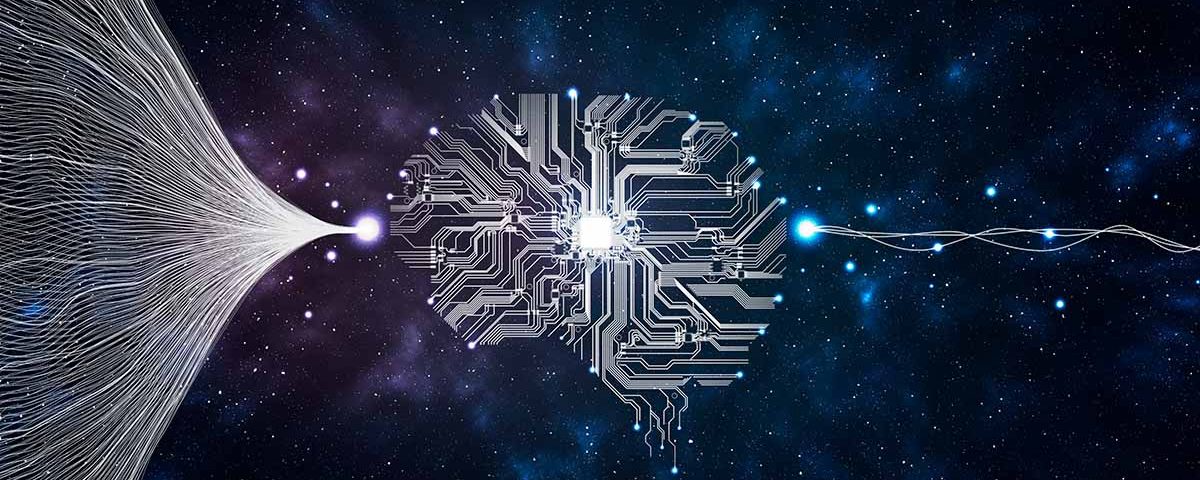
Over the last few years, which have been shaped by Covid, significant advances in artificial intelligence have been made. Expectations have not ceased to be exceeded in many regards. Many companies have surpassed the stage where AI was mere experimentation, and they have managed to sharpen their competitive edge with this technology.
Ferrovial is among these cases; since 2016, the company has been working on various artificial intelligence initiatives that have enabled it to generate efficiencies and new revenue and to reduce the risks associated with its activity.
After all, for AI to have a real impact, it must meet a business need, and that can’t be done without having business members on the work teams.
Now, what’s all this about artificial intelligence in business?
Artificial intelligence can get mixed up with data science or even statistics in a company because the goal is not the technology itself but the value it provides. That is why the term is often used to encompass any process where data (whether big or small) and some algorithms (whether from statistics, machine learning, neural networks…) are used to obtain information (which, as we’ll see, is a very broad concept) which would not have been gotten otherwise.
What’s this about information?
It takes many forms, but there’s only one goal: creating and optimizing business processes.
Some forms of information we obtain here at the DigitalHub are:
- macro trends in the price of building materials
- detecting defects in electrical facilities
- vehicle demand prediction for carsharing services like Zity
- parking rate optimization
- picking up passengers at airports after the pandemic
- report categorization
There are many more examples, but we can get an idea of the variety in this information.
And what about data?
Data has been with us for a long time: never-ending Excel spreadsheets, report after report on performance, tenders, technical requirements…
Later on, data came from machines: acceleration, velocity, consumption, and endless data on the operation of excavating machines, cars…
And there was data on temperature, energy consumption at facilities…
Then, there was the data we didn’t produce but that other companies or public entities gave us: investment data from different governments, climate, economic, demographic data…
And they were all so different! They were all in different places!
This is why we created the Data Lake. A space that allows us to keep all the data in one place and in the most standardized and automated way possible, so that we could always access ready-to-process data from one single place. It was important for this data to be adequately governed and managed in order to facilitate its use, increase its quality, avoid risks and guarantee its ethical treatment.
In complex organizations like Ferrovial, several Data Lakes were created which also had to be known, accessible and coordinated through a common governance structure.
How do we get from data to information?
We transform data into information through algorithms, and we can do so in various ways. These could be classified by what is learned:
- Predictive algorithms: these algorithms are used to learn how output data is generated from input data.
One example can be seen in a field’s daily water demand (output data), which can be understood in relation to the time of year, the types of cultivation, each’s water demand… Then, the future demand can be predicted, and you can prepare for it.
Another good example is when a user leaves a certain app. Average daily usage data, average weekly usage, other apps… All this data can help us understand why a user might leave an app in order to predict when other users will leave us – and act before that happens.
- Grouping algorithms: in this case, we don’t have an output variable; instead, we aim to group different pieces of data based on their similarity. Each type of data has its own measure of similarity, normally given by a distance, and we’ll group the data that is closest together.
An example of this type of data is spatial groupings: if we want to carry out a promotion for works involving building restoration, we’ll want to define the areas where our action may be most beneficial: grouping buildings with the worst energy rating, those with the worst paved streets… and come up with an offer tailored to each similar area, without having to specify for each and every building in the city.
Another good example is grouping users based on how they behave: car rentals, hours per rental… you can make personalized offers through these groups, thereby increasing your rentals.
However, information is not only the outcome of a model applied to data. Information has a lot to do with the meaning given to what comes out of the model (some predictions). Without business, these don’t have any meaning.
That is why the work always has to involve the person who will use it: to help them optimize their processes.
AI is dead, long live AI!
At the beginning of this post, we compared AI with a technology as basic and transformational as electricity. Electricity took more than half a century to have a real impact on companies, and not because of the technology itself but because of the need for companies and society as a whole to find the best way to harness it. Transforming production lines, factories, lighting, etc. was necessary, but the outcome of that shift is now evident.
Something similar is happening with artificial intelligence. At times, it is being incorporated into processes more slowly than we might expect, but that has more to do with companies’ ability to reinvent their processes in order to extract the maximum value from technology than with AI itself.
Moreover, like every basic technology, the trend for AI as it penetrates all sectors is to disappear.
Nowadays, we don’t think about electricity (besides how to look for new sources, storage, and more sustainable transmission), and the term “artificial intelligence” is showing similar signs of disappearance. We talk about self-driving vehicles and robots, and we often forget that artificial intelligence is precisely what gives those devices autonomy. We talk about Industry 4.0 and digital twins without thinking about how AI is what imbues these terms with real utility in advanced control scenarios, simulation, prediction, and autonomous reaction to situations.
Finally, to drop another in-vogue term, we also talk about metaverses, digital universes where we interact with environments, objects, and entities whose behavior is largely defined by Artificial Intelligence.
In conclusion, the less we hear the term “artificial intelligence,” the more likely its impact will be greater. That’s why we say, “AI is dead, long live AI!”
There are no comments yet