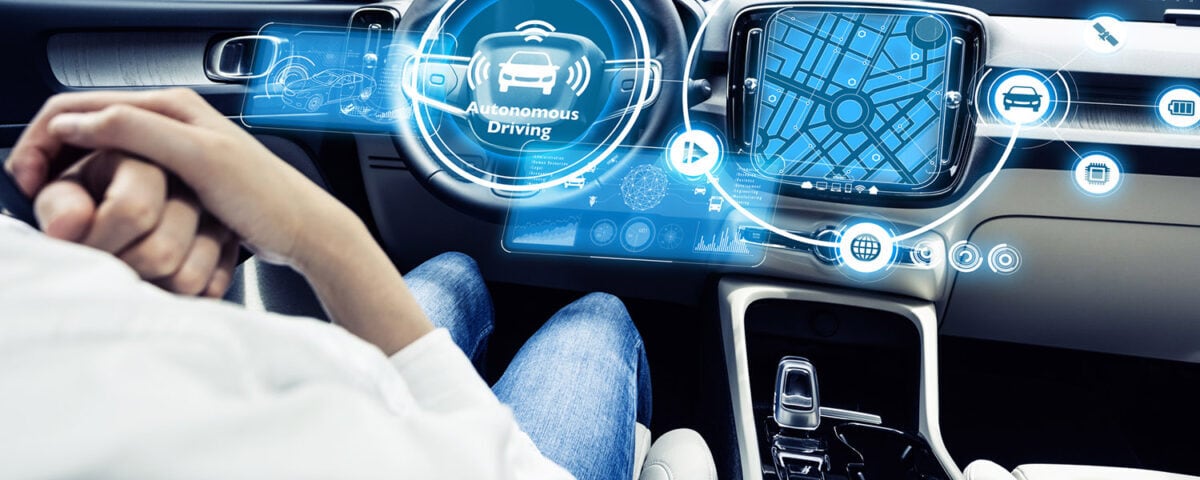
Since Mercedes-Benz and the University of Munich developed the first autonomous vehicle (AV) in the 1980s, enormous efforts have been devoted to this technology. While AVs are often judged regarding their implications, benefits, development, and technological challenges, less attention has been paid to users’ acceptance of their usefulness. In this blog, I’d like to go over the aspects that influence the general public’s perception of AVs, focusing on the implications that the current degree of technological development has on issues such as security, ethics, legal responsibility, and legislation, and how the latter influence users’ acceptance of AVs.
Safety in AVs
While AVs have the potential to increase traffic safety due to preventing the human factor (human error causes over 90% of accidents), there is still the possibility for the vehicle to fail for a wide range of reasons, such as extreme environmental conditions or more generally, the wide variety of situations and scenarios where vehicles not considered in advance may be involved. These reasons make getting the AV to behave safely in any situation a highly sophisticated process that requires a high level of cooperation and coordination between multiple disciplines, from artificial intelligence and machine learning to sensor systems and test procedures. On this point, it’s interesting to discuss the level of AVs’ technological development as a support to understand its safety implications.
Technology used in AVs: sensor fusion, computer vision, and fault tolerance
- Perception
Although object perception is a difficult task, engineers have developed systems based on diversity (different types of sensors) and redundancy (duplicity of sensors) to optimize the accuracy of measurements and ensure operation in the event of failures. To provide the situational information necessary to operate safely, three types of sensors are typically used: camera, radar, and lidar. While cameras are the best form of visualization, they have their own limitations. A camera cannot calculate the distance to objects accurately, and in many cases, it’s hard for them to detect objects in low visibility conditions, such as fog, rain, snow, or at night. Radars help to strengthen camera detection in low visibility conditions and measure speeds and distances more accurately. In fact, most conventional cars today have cameras and radar sensors in their driving assistance, parking assistance, and other systems.
However, the information provided by cameras and radars is not enough for a vehicle to operate autonomously; therefore, the system is reinforced with lidar sensors, which can measure distances ultra-precisely. This technology is based on the use of pulsing lasers that make it possible to construct 3D images with very high definition. In addition, developers use all three types of sensors at once to improve perception through a process called sensor fusion.
Image of the point cloud generated by a lidar
Sensor fusion can be defined as the process of combining data from different sensors to improve the performance and accuracy of the vehicle in a way that cannot be achieved with a single sensor. During the fusion process, inputs from the sensors are fed into a central computer with a large processing capacity that combines the data and executes decision-making. By using the information from the set of sensors, the system becomes resistant to any failures of the individual sensors and also provides redundancy since the same area is covered by different sensors, thus improving decision-making.
- Decision-making
While detection is an important task, AVs must interpret their environment to make decisions. Manufacturers use artificial intelligence and machine learning to support this decision-making. These technologies are based on training the system with a massive amount of data and situations.
- Navigation
In a simplified way, the navigation process is a succession of the vehicle’s spatial position in time. The vehicle’s position at each instant is defined by a set of parameters that constitute what are known as system states. Navigating a vehicle consists of estimating its condition over time. In general, state estimation methods are based on control theory, which employs the laws of probability to calculate a state vector from a vector of measures. There are three status estimation methods used in autonomous driving: machine learning, the Kalman filter, and the particle filter.
- Artificial vision
The image processing algorithms required for an AV to operate safely are extremely complex. The AV must also be equipped with the necessary power to process onboard images so that the vehicle can react to the world around it and avoid objects. Another challenge is the hardware platform required for image processing. It must be sufficiently light but at the same time be capable of providing the processing power required, with low consumption and the capability to operate robustly for a long time.
- Fault tolerance
The period when data is delivered in a faulty state is called a service interruption period. There are four main approaches to avoiding periods of service interruption according to how system failures are treated: prevention, elimination, prediction, and tolerance. While fault prevention and elimination focus on preventing or eliminating failures, predictive and fault tolerance approaches are based on keeping the operating system going in the presence of failures and avoiding unplanned behavior.
For extremely complex processes, it is not always possible to implement a fault-free control system, so prediction and fault tolerance approaches are the preferred approaches. For AVs, the fault tolerance approach is the most widely used.
The main objective of Fault Tolerant Control (FTC) is to detect faults efficiently early on and allow the system to operate conveniently even with the presence of any faults. Mathematical models are widely used in FTC systems because their parameters can generally be easily obtained. However, for complex tasks, it is difficult to develop an accurate, representative mathematical model of the system, or it might not even be possible. In such cases, other techniques, such as neural networks, are used for detecting and diagnosing faults.
After the error is identified, two methods are used for fault recovery: direct redundancy or analytical redundancy. In the former, an additional module is used to replace the defective component. Analytical redundancy, on the other hand, involves using the rest of the modules that work to complement the tasks of those that have failed.
The ethical problem
In addition to safety, there are other aspects that impact the general public’s acceptance of using AVs. AVs are up against an ethical dilemma similar to the famous tram paradox; however, in the case of AVs, this paradox occurs continuously. The main problem is that, unlike human drivers, an AV’s decision on how to behave is predefined by a programmer. This dilemma has a significant impact on AVs’ acceptance.
Legal responsibility of AVs
Like safety and ethical issues, legal responsibility and legislation have a significant, if not critical, impact on AV acceptance. One of the main challenges facing the AV’s introduction is the need for changing laws to adapt to autonomous driving. And the key question is: can the AV drive? One of the fundamental benefits of autonomous driving is allowing human drivers to participate in other activities besides driving during their trip. The main problem is that most countries consider the human driver solely responsible in the event of an error or accident. Therefore, if the law doesn’t relieve them of this responsibility and the driver is required to keep their attention on the road while the car is moving, then what’s the difference between AVs and conventional vehicles? In that case, will people agree to buy such a vehicle? Apparently, as in many other technology-related cases, regulation is lagging behind AV development rather than taking the initiative.
Other factors to consider: The COVID-19 pandemic
AVs have the potential to help people fight pandemics. AVs have been used to transport people and deliver food and medicine during the lockdown due to the COVID-19 pandemic; this has led to increased interest in autonomous driving systems, opened consumers’ eyes to the benefits of AVs, and in turn, increased public support for them.
Observations and recommendations for the future
Safety: The safety of autonomous cars is paramount. If the vehicles are not safe, they are significantly less desirable, regardless of their benefits. AVs’ perceived safety, or rather their perceived lack of safety, is what will really sway potential buyers’ opinions. That said, manufacturers should put greater emphasis on the safety systems they incorporate into their vehicles and show the public that using this type of vehicle is risk-free.
Accountability: The industry must force comprehensive, reasonable laws for self-driving cars to be developed. Only when AVs are totally safe and the laws regarding them are satisfactory will people judge whether the cost of these vehicles is worth it or not.
Ethical dilemma: One of the main problems of AVs is that, unlike human driving, the AV’s decisions on how to behave in an extreme situation are predefined by a programmer. Currently, the way this decision-making is designed or assigned is an unresolved problem, and more studies are needed to make the appropriate decision in similar situations and understand the social acceptance of various alternatives. These concerns have a significant impact on public acceptance of AVs, and if they are not resolved, the price of the vehicle is irrelevant.
There are no comments yet