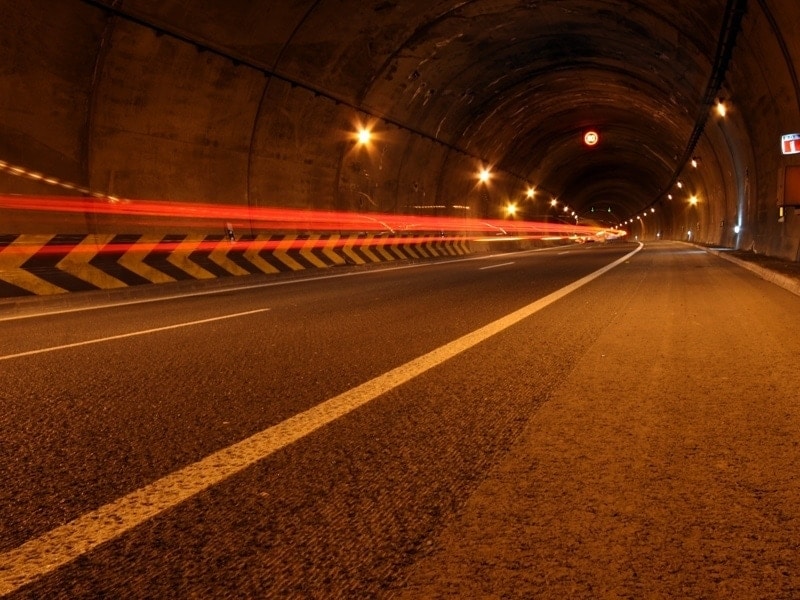
When talking about digital transformation, one of the concepts we always seem to associate with it is “Big Data”. In general, Big Data is understood as being a new technology which allows us to analyse large amounts of data, although it is also one of the processes resulting from rapid technological acceleration that creates the greatest uncertainty. So statements such as… “Big Data is like teenage sex: everyone talks about it, nobody really knows how to do it, everyone thinks everyone else is doing it, so everyone claims they are doing it…” have sometimes appeared on different social networks. One very common question in this area is: where does the availability of such large volumes of data take us?
Many startups are being created today to take advantage of data and data interpretation as an opportunity to improve processes and offer solutions to make corporate decisions. This is the case of Kineo Mobility Analytics, a company with which Ferrovial and Cintra are developing a project for modelling demand on toll roads. The project analyses large volumes of geolocalised data to find out more about user needs. We interviewed Ricardo Herranz, cofounder of Kineo, to find out exactly what it is that Kineo does and what projects they are working on.
Interview with Ricardo Herranz, cofounder of Kineo
- Ricardo, what does Big Data mean to you?
Everyone has been talking about Big Data for some time now. My feeling is that there is no clear consensus on what it really means. Generally, Big Data could be defined as the handling and analysis of large amounts of data which it would not be possible to treat with conventional tools and methodologies, but overuse of the term has meant that it is often taken as a synonym for more traditional terms, such as Business Intelligence or Data Analytics, or, more recently (and possibly more pretentiously), Data Science.
All of these concepts are linked to transforming data into information that can be used for decision-making. The most obvious difference between Big Data and traditional analysis is the volume of data handled. The amount of data we generate increases day by day, but so does our capacity for storage and processing. Articles on the subject very often state things like “such and such percentage of world data has been generated in the last year alone”. Another perhaps more relevant characteristic is that the data stems from all sorts of diverse and very different sources, in a very unstructured way, and that such data is frequently used for a purpose different to that for which it was generated and registered in the first place. This is the case with data sourced from Internet social networks: here we have data which is enormously useful for studying human behaviour, for example, but which comes with a lot of “noise” and significant biases. And so it becomes necessary to develop mathematical models and methodologies for analysis that allow filtering, correcting and merging of data from different sources to extract useful and reliable information.
- Based on your professional experience – more than 15 years in research and as a consultant in data analysis projects –, do you believe that the use of new technologies has greatly changed the way we access and interpret information?
It seems very obvious that new technologies have radically changed access to information, and probably also the way in which we digest and interpret such information. Web 2.0 has turned us not only into information consumers, but also into information producers. But such information, as I said before, is full of noise and bias. We don’t all have the same level of access to data. And neither the data nor the algorithms are “neutral”, starting right from the moment when we decide what information to collect. If we fail to understand the limitations of all this data and the algorithms required for its critical interpretation, what we have is a false sense of objectivity and of democratisation of information. There are many interesting discussions to be had as to what actually separates the use of data as a means to empowerment, and the use of data as tool of power, – a distinction that is sometimes rather blurred–. But that would no doubt be the subject of a different blog.
From a scientific perspective, the availability of large amounts of data and the emergence of tools capable of managing such data open up new opportunities for addressing issues which were well beyond the reach of traditional quantitative analysis, particularly in the field of social sciences. But I believe we have not yet succeeded in developing a conceptual framework for getting the most out of this wealth of data. In the same way that mathematical models are practically useless if there is no reliable data for weighting and validating them, data is little more than a collection of numbers if we have no models with which to interpret such numbers. Just as much as we have to be able to formulate a problem in mathematical terms, we need the capacity to interpret the results of models developed in terms of the initial problem. I believe that those of us using Big Data in research and consulting must do so credibly and responsibly, recognising the need for an interconnected and multidisciplinary perspective. And that is what we try to do, to the best of our ability, at Kineo.
- What type of solutions does Kineo Mobility Analytics provide? Or to put it another way, what type of technology do you use to obtain information, and how do you interpret it?
Kineo analyses anonymous geolocalised data from mobile devices to supply private enterprises and public institutions with useful information on the activity and mobility of the population. What we do is collect and analyse mobile telephone and other geolocalised data from various sources (smart cards, GPS, Internet-based social networks, etc.) and merge these with more conventional data (such as census information) to provide answers to questions such as, for example, how many people converge on certain locations at different times during the day, what type of activity they carry out, how long they do it for, their economic and social profile, types of transport used, etc.
The ability to classify people’s activity and mobility in a dynamic way at a significantly lower cost than using traditional methods opens up a wealth of applications in fields such as town planning, transport, tourism, environment or public health policy development, amongst others. In the case of Kineo, the provision of such information is complemented with our modelling and simulation capacity, which allows us to offer value added services (such as traffic and income forecasts for toll motorways, as in the case of our joint project with Ferrovial and Cintra), thus helping the client to maximise value from data.
- Why is geolocalisation such a valuable source of information?
This might seem obvious, but everything happens somewhere. Concepts such as distance and spatial interaction are at the forefront of territorial planning, and spatial structures in turn determine what processes will take place in them. Planning and evaluation of public policies, defining the size and scale of infrastructures, and managing a large diversity of services requires the data, models and tools with which to understand and predict people’s behaviour in terms of spatial patterns.
The sources of traditional data – basically surveys – provide valuable information, but they also have significant drawbacks. Surveys are expensive and slow to carry out, and this restricts the size of samples surveyed and the frequency with which information is updated. Intrinsic limitations such as incorrect or imprecise replies, or the willingness of respondents to take part, add to the drawbacks. With today’s massive influx of information and communication technologies, practically all our daily activities leave behind a digital geolocalised footprint, generating a massive amount of data – with a spatial and temporal resolution never before possible – which can help us address a large variety of economic and social problems.
- What amount and what type of data can we generate from a simple car trip? Why is this information important for our clients?
A car trip generates several types of geolocalised data: the succession of grid cells from the mobile phone network we are at every time our phone connects to the network allows us to track our path; our phone use patterns provide information on the purpose of the trip. Our path can also be tracked, in a much more precise manner, if we can track the GPS position of our mobile phone by using a specific app, or from the route registered on the GPS car navigation system. Analysis of data captured by other mobile phone sensors, such as accelerometers, allows us to identify the type of transport used for the trip, the car in this case, as opposed to other types of transport such as the train. When the car passes certain control points it will be registered through vehicle counting systems, such as spires or cameras, or by electronic toll collecting systems. By merging and analysing this data we can track demand patterns, which are crucial for planning infrastructures, urban public transport systems, or the charging strategy for a toll road, to give just a few examples.
Application of all of this technology to the transport and mobility sector is perhaps the most obvious use, but it is not the only sector where this information is valuable. Tourist movements and the areas they visit; the number of people exposed to air pollution or certain noise levels and their sociodemographic background; where the customers of a certain shopping outlet come from and the routes they travel to get there… There are numerous sectors where geolocalised information improves decision-making and thus results in a better service for the end user.
- What do you gain from working with a company such as Ferrovial? What experience have you obtained from the project?
On the one hand, working with Ferrovial and Cintra is allowing us to validate the application of our solutions in a real-life environment and fine-tune our product and services portfolio in the toll road sector. The project has a significant experimental and learning component for both sides: we are learning to better understand the needs of a client such as Cintra, and we believe that Ferrovial – Cintra innovation team is learning to understand the value of the information that Kineo can provide, and getting to know how to make the best use of that information. Both communications and knowledge-sharing with the Ferrovial-Cintra team and project results are proving highly satisfactory, and we are confident that this will be just one of many successful joint experiences.
On the other hand, working with a world leader in infrastructure management also has its reward in terms of reputation and credibility. In an emerging market where confidence-building is still critical, projects such as the one we are carrying out with Ferrovial-Cintra allow us to prove the worth of our proposals and break down barriers for the adoption of our solutions by other more conservative clients, or those with a lower innovation capacity.
- And now a bit of a cliché question… but we cannot end the interview without it: as an entrepreneur, what advice would you give to other people who are thinking of creating their own startups?
I don’t feel very comfortable giving advice, but still… I’ll also start with something of a cliché. My first piece of advice would be to surround yourself with good people, people you feel comfortable with. This is something that requires time (sometimes a lot of time), but it is essential that you find the best possible partners, staff and colleagues. The second bit of advice would be to concentrate specifically on creating and transmitting a strong organisational culture, promoting team work, a commitment to the company and to the client, and personal career development. Thirdly, use every opportunity you can to cooperate and generate shared value with all the actors in the value chain. As a last bit of advice, and since we are talking of Big Data, make sure that you filter out the noise and the bias from all the advice you will no doubt be receiving from people who are as little suited to giving advice as I am.
There are no comments yet