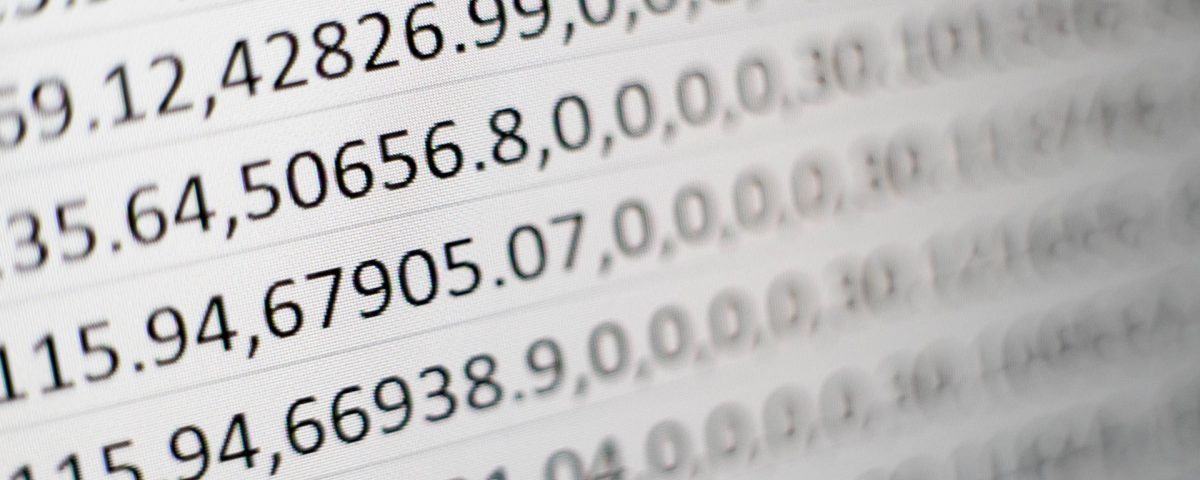
A data scientist’s work is often perceived as some sort of magic through a computer. Maybe they can predict the future with data, too. The truth is, when it comes to thinking about our work, the focus is always on the person who carries out the task when it should be on the data itself.
A data scientist is a professional whose main role is identifying patterns or extracting knowledge from data by using algorithms for analysis or through building mathematical models. Once this is done, they interpret the results to draw logical conclusions and predict future behaviors. Based on this information, stakeholders can make decisions and choose where to direct their lines of business.
However, sometimes the impossible is asked of us. The data is expected to justify certain aims that have already been determined, or to sketch a reality that, though it may be ideal for the business, does not always actually exist. We can’t do magic with data, nor can we transform it to give the results we want.
The truth is, in our daily work, we look at data without prejudice and treat it for what it is: an essential source of information for decision-making. These are the three main principles that must be taken into account to understand how data scientists work:
Let the data speak for itself
Often, we jump to conclusions about the patterns that we’ll obtain. We do this even before starting to work with the data itself. This information can show us realities we didn’t know about, and that is why we have to have an open mind and never just go with our gut.
When the results contradict logical hypotheses, we must ask ourselves if there is a reasonable explanation. Other interesting questions that may arise include: how was the data obtained? Does the algorithm used make sense, and is the approach the right one for the problem?
We data scientists look at data without prejudice. However, we frequently have to clean up data before starting. This is because we can find erroneous data (due to failures in the sensors that collect them, for instance), embellishments (which are intentionally introduced to favor certain results), or biases (which condition how the information was obtained).
All models are wrong, but some are useful.
This saying attributed to George E.P. Box highlights the fact that there is no universal model that makes sense for all data. Our world contains countless models that can be applied. A fundamental part of our work is identifying which algorithms best fit each case.
An important nuance to bear in mind is that each model makes assumptions about the data we use. When the data (which is numeric) doesn’t fit a specific model, we can transform it so that it does fit. In other cases, we can choose another less restrictive model.
This is where our experience and ability to test new solutions come into play. Even if we know a model can work, we must not rule out other possible candidates. Again, it is essential to avoid biases.
Data quality, the key to success
The quality of the data largely determines the quality of a project’s results. Good data makes it possible to make decent predictions , even with models that aren’t fully compatible with the information we have. However, when the data is of poor quality, even the most sophisticated model can fail in its predictions. It’s like trying to build a wooden house with beams infested with termites. The house will fall down. That’s why we hear that data is the oil of the 21st century – the tools for companies to define their strategies.
It is ideal to have large volumes of information, though there are exceptions where small amounts of data will work. It’s also advisable to have rich, varied data and to avoid redundant, erroneous information.
If those three conditions are met, we data scientists can do our job. This will allow a brave stakeholder working in a decision-making culture based on that work can do things that seem like magic.
In other words, models and patterns are worthless without the data feeding them. The conclusions drawn from data are what have real strategic value. This is why I encourage you to curate your data as much as possible. That data is a commitment to the future.
There are no comments yet