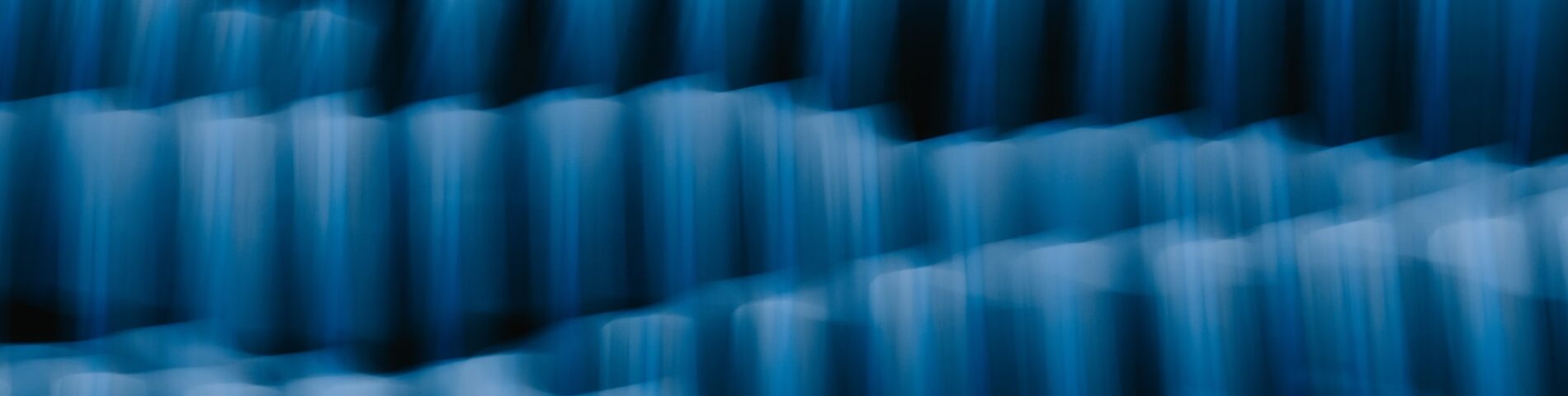
How sound and artificial intelligence are used in machine engineering
15 of June of 2022
Artificial intelligence is rapidly making its way into the industry. It’s long been used to design better materials and optimize traffic flows in cities. Some of its features and applications are fascinating, as is the case with artificial intelligence that listens to detect anomalies in engine tests.
The importance of sound in engineering
Have you ever heard a sound like marbles or bearings rolling across your upstairs neighbor’s floor? This is due to the ‘water hammer’ phenomenon where the pressure rises noticeably and abruptly in the pipes. Alongside water hammering, cavitation is the main cause of breakdowns in hydraulic installations. Both cause significant damage to the systems.
This phenomenon causes steam bubbles to form, which not only wear away the pipes but also on machines like pumps or parts like blades. In fact, the cavitation phenomenon can destroy generator blades in a hydroelectric power plant or the propellers of submarines and ships. It can go so far as to pierce stainless steel and twist it out of shape.
Cavitation generates high-intensity micro-explosions with wave velocities similar to those of sound. Needless to say, it has been studied to find ways to prevent it. In this regard, the study of sound is indispensable, just like when an engine is checked out at your neighborhood auto shop.
A good mechanic listens to what a car sounds like to determine the diagnosis with a good degree of accuracy. However, this experience is limited if there’s a wide variety of engines or components. As complexity increases or quality becomes a more important criterion, the human ear is less and less accurate. That’s where machines come to the fore.
Tractian, the Shazam of Engineering
Engineers have been developing systems that listen better than people for several decades, so much so that they can anticipate machine failures. This is the case with Tractian, the so-called “Shazam of Engineering.” At this company, they can diagnose errors by active listening with microphones. Machines that diagnose machines.
As managers note in an interview, listening with Tractian can predict when a machine might fail, but it’s not a crystal ball. Since the 18th century, engineers have been pinpointing when a part might fail in fairly significant detail. The stress-strain curve is one example of this applied to materials.
This curve is performed by testing the material tens, hundreds, or thousands of times, noting the characteristic curve and when it becomes a fracture (upper right). This was called ‘material characterization’, and it is the basis of predictive machine maintenance, albeit with an obvious increase in complexity.
If you know how many cycles it takes to break a turbine or pump, a warning when it reaches 95% of that amount can be crucial. But it’s even more useful to be able to detect what acoustic-vibrational signals a machine puts out before failing. Therein lies the key to accurate, predictive systems. That’s exactly what they do at Tractian with their new program.
Getting ahead of the breakdown also makes it possible to prevent others. If listening to a machine can allow it to be repaired before that error causes further deterioration, that extends the life of the device, thus lowering its cost and its environmental impact. That’s like taking it in to the shop when the engine sounds ‘weird,’ instead of driving until the car breaks down and the entire engine has to be changed.
How Sounce, the AI system that could revolutionize engineering, works
In this regard, luxury vehicle manufacturer Porsche launched a virtual assistant called Sounce in 2020 to monitor sound during testing on vehicle parts. This system was not limited to collecting information like ‘material characterization.’ Sounce learns through machine learning techniques and can infer new errors to detect even when people cannot.
To date, Sounce has ‘only’ worked in the design and manufacturing phases of various parts in factories. This in and of itself entails a significant achievement when it comes to minimizing design and manufacturing errors. Thanks to the system’s artificial intelligence, the assembly process and test benches are much safer and more reliable. But why?
Because this system audits a very basic process of vehicle production. Instead of picking up sounds once the car is running (which will be the next phase), it monitors the machines that make the parts, and the parts themselves, before the vehicle hits the road. Errors are detected earlier, so it’s possible to take action at the factory to increase the quality of the entire process.
The fact that they use artificial intelligence and machine learning techniques makes the model learn over time, so this noise detection can be said to be getting better every day. In the future, it’s likely to be in all vehicles.
Noise monitoring in uncontrolled environments
Unlike a factory, a road is not what you’d call a controlled environment. A vehicle could tip over, drive the wrong direction on a one-way road, or simply stop for its occupant to get out right in the middle of the lane. Human errors and accidents are a reality. That’s why it is so interesting for technology like AI listening to be implemented in vehicles: it will prevent accidents.
Listening technology to anticipate machine breakdowns will not only be useful on the road. Systems like airplanes, large ships, or trains will also be able to set up new early warning systems. What if a jet plane explosion could be detected before take-off, or a train derailing could be foreseen by how the track vibrates ten kilometers before it happens?
Of course, this doesn’t just apply to machines. Large structures like aqueducts, bridges, dams, tunnels, and other infrastructure may benefit from these systems in the future, just as they already use elements like strain gauges to measure micro-displacements that warn of minute deformations.
There are no comments yet