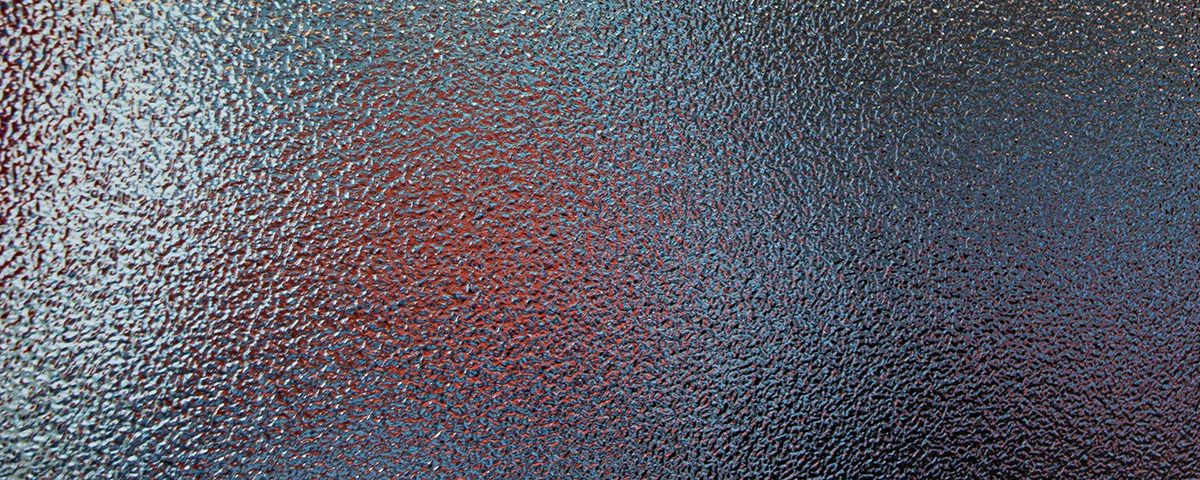
Could artificial intelligence help us design new materials? In mid-2018, a promising article was published in the journal Science Advances. A team of researchers had discovered metallic glasses in an “accelerated manner.” To do so, they had used machine learning.
One of the biggest challenges of materials engineering is finding the right substance for a certain purpose based on certain properties the material must have. For example, what material could replace concrete? How can we design more sustainable asphalt?
As the authors noted in the abstract, “searching the vast combinatorial space [of material combinations] is frustratingly slow and expensive,” and a new way of finding suitable materials is needed. That new way is artificial intelligence.
How can artificial intelligence find new materials?
Every existing material can be virtualized as a data set. Alloy steel, for instance, is made from specific proportions of iron and carbon (along with other elements) that undergo a specific process and take on a certain structure. That is, there’s a ‘chemical composition’ that goes with a certain internal organization and manufacturing process.
This data set gives rise to a different set of properties that can be called ‘material properties.’ These include viscosity, melting point, compressive strength, fluidity, conductivity, etc. Material properties are a function of composition, or in other words, each composition will give rise to a set of properties.
properties = f(composition)
Steel | % carbon | Tensile strength (kgf/mm2) |
Extra-mild | 0.1 to 0.2 | 35 |
Mild | 0.2 to 0.3 | 45 |
Semi-mild | 0.3 to 0.4 | 55 |
Medium-hard | 0.4 to 0.5 | 65 |
Hard | 0.5 to 0.6 | 75 |
Extra-hard | 0.6 to 0.7 | 85 |
Material characterization, which is the study of their properties, has been generating tables like the one shown above for centuries. It shows the tensile strength of the steel based on the percentage of carbon over iron. Each percentage of carbon translates into a different resistance.
However, these tables are quite limited. Does a semi-mild steel with 0.31% carbon have the same strength as one with 0.39% carbon? Obviously not. It’s not a discrete function but a continuous one. The problem is that obtaining each discrete piece of information is expensive because the material has to be manufactured and tested. That’s why tables are tables and not formulas.
For several centuries, statistics have been filling in the unknown gaps by using interpolation techniques – for example, 0.2% steel must be between 35 and 45 kg/mm2, so about 40 kg/mm2 is an allowable figure. But as new materials emerge and we reach their limits, testing becomes more and more expensive.
That’s where artificial intelligence comes in. It can ‘fill in’ gaps in tables with materials that have never been produced, predicting properties based on what is known about other materials and even developing new tables on its own.
The AI that took two hours to build the periodic table
The periodic table is one of humankind’s greatest achievements when it comes to contextualizing matter. In 1869, Russian chemist Dmitri Mendeleev presented this table to the Russian Chemical Society, and it has evolved over the years as new elements were discovered.
Dmitri Mendeleev’s Periodic Table (1871)
He accomplished something incredible with this table. Not only did he manage to order known matter, but he could also make predictions about elements that weren’t yet known based on the unoccupied chemical properties in the table. The first attempts at a periodic table date back to 1780, and it has taken us almost 250 years to shape and polish it.
That’s why it is so fascinating to watch artificial intelligence like Atom2Vec sort out a list of chemical compounds and draw a periodic table with the basic properties of each element on its own in two hours. Furthermore, it did this in a vector space (which humans can’t read without tools) rather than the table we’re used to seeing.
What has been achieved so far with ‘AI materials?’
We began the article by mentioning a publication about new types of metallic glass that were discovered using machine learning. To understand its potential, just consider that, for the 6,000 new combinations tested to form different metallic glasses over the last 50 years, some 20,000 permutations were tested during the first year of machine learning.
The possibilities that artificial intelligence offers are immense. Recent years have seen the discovery of new hybrids of glass and metal, new properties of two-dimensional materials like graphene, new electrolytes for electric batteries, and the analysis of new silicon-germanium structures that work better thermally than current ones.
The list keeps growing every day, and this field has long ceased to be confined to the lab. The new aluminum alloys are already being used in applications like automobiles and pressure vessels. It’s only been a couple of years since machine learning tools started ‘combing’ the PoLyInfo database, but tens of thousands of promising lines of research have already been identified.
There’s no doubt that artificial intelligence is going to transform the way we find new materials, which will, in turn, lead to new challenges. If the biggest difficulty in the time before AI was in the cost of testing materials and determining their properties, the challenges of the AI era are computing power, data quality, and filtering information.
There are no comments yet