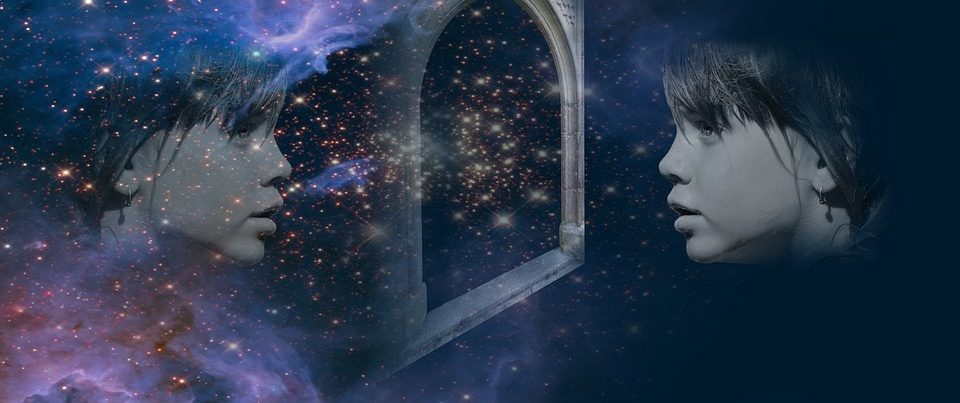
The concept of the digital twin is the hot topic of the day, and it can be heard at hundreds of talks, forums, and events where Industry 4.0 is the main subject. Similarly, there are hundreds of definitions and writings that try to explain, and even sell, what a digital twin is or why it should be the basis of the industrial research that every production plant must develop. However, the reflections described here will try to get us up to speed on what it looks like day-to-day and how using this technology has actually improved manufacturing production processes.
Setting aside the technicalities or dictionary definitions of the words “Digital” and “Twin,” as well as whether it was NASA that coined the term a few years ago, we will describe in just a few words the concept of the Digital Twin. Specifically, it is a virtual, intangible representation that tries to reproduce a physical or real situation, like that carried out in a manufacturing process. The fundamental help provided by the twin is decoupling the physical world from the virtual one and being able to work in that fantastic, magical world so that in the physical world, no unanticipated deviations arise.
In other words, the digital twin has to provide improvement for the process. In the case of manufacturing processes, interests are usually focused on defect reduction, energy improvements, or time-to-market reductions. And this must be accomplished under three clear axioms of operation:
- Proactivity. The system must be able to anticipate adverse situations in production. It must use the digital world so that, with simulations and Artificial Intelligence, the situation in which the production process will be in a future temporal state can be determined.
- Setting. The digital system must always work within a specific configuration of the manufacturing process. That way, all simulations, checks, and tests developed in the digital realm will obey the rules governing the physical or real representation of the process.
- Business intelligence. The twin is a global repository of business intelligence. That intelligence will be used to perform its tasks, but what is important is the maintenance and management of that intelligence within the plant itself. That is, in addition to helping us in improving the process, a digital twin is a tool for managing knowledge for its generation and storage.
The whole theory is really nice, but the key question is: how? The answer is simple. The way to achieve it is based on the methodology carried out by doctors called “diagnosis.” There are three basic steps: (i) observation, (ii) evaluation, and (iii) decision-making.
Observation is the basic need for the digital twin
The fundamental basis of improving a production process is knowing it as much as possible. So, in addition to having experts in that process, it is necessary to have clear, extensive information about what is happening in it. This is where the observation process begins. Continuing with the analogy used before, doctors are responsible for making observations through their hands, eyes, and measuring devices. Thanks to them, they can gather the patient’s current reality, and that is the basis for their diagnostic work.
Likewise, the digital twin, working as an expert doctor, needs to extract the information, but in a slightly different way. Its observation is based on raw data that can be subsequently analyzed through advanced Data Analytics techniques. This data comes from the twin’s communication with the production process databases, the extraction of real-time information from the sensor network deployed throughout the production process (which today is called the Industrial Internet of Things), or other systems deployed in the plant for collecting information associated with production orders, clients, or work stages, among others.
This entire collection of data is what will allow the virtual system to work in two clear ways. The first of these is the primary one and the basis of everything. It is one on which the entire twin will be supported, and it is from this data that the operating patterns, trends, and other aspects will be extracted, which affect the second one, the prediction or simulation of situations that could occur in the real world. Also, observation will be useful for enabling working with the current state of the production process and trying to optimize it.
We should keep in mind that, without observation, that is, without gathering data, there is no way to develop such technologies. It is highly likely that the first step to take is setting up some kind of project that will allow our plants to move forward at this level. Once this operation of observation is resolved, it is time to move forward in developing this type of digital solutions.
The operations that have to be carried out will be dependent on the evaluations done
Once the twin is loaded with the knowledge generated from observing a wide range of historical and the current real-time data of what is happening, the twin starts working right away. This process is known as “Evaluation,” and this is when the digital twin begins to run different simulations with the reality that it is receiving through observation. This is where the twin makes uses of advanced prediction and classification techniques based on AI in order to anticipate what might happen at the plant.
Developing and combining different methods is necessary for accomplishing this evaluation task. That way, there are first simulations based on classic simulation methods that make the use of formulas that model physical behavior in the real world. There are also methods based on expert knowledge taken from the process and from the workers. This technique is a quick, simple way to use a series of rules and patterns that enable obtaining those assessments. This technique is based on the old Artificial Intelligence systems that might seem obsolete, but in the case of production processes, there are certain very simple evaluations that do not need to be complicated with other types of models. Finally, there is the possibility of using advanced techniques for statistical classification or clustering. It is these that are abuzz among researchers and that have names like Machine Learning, Deep Learning, Neural Networks, and so on. Although generating these models or even just understanding them may be complicated, their goal is to be able to provide a future value based on previous cases and how the process behaved in those situations. The systems make use of different techniques that enable showing the information used by the algorithm, and finally, when new input is available (the real-time observation), it is compared to historical data to determine the state that would be reached.
This stage is one of the most complex, and it receives the most attention because it is responsible for making the magic of digital twins. This is the stage where the digital twin looks into its crystal ball to determine those future situations and get ahead of reality. However, as mentioned, the models that have to provide content during the evaluation process cannot be generated without knowledge and data. We can then see that observation is the fundamental stage for a digital twin’s proper functioning.
A machine that makes decisions on process optimization
Just one observation will not give the production process an edge. It’s true that we will have greater control because we’ll have everything that happens in our process recorded in real-time, but data alone will not improve the process. Similarly, the evaluation process is very interesting, but knowing that we will be in an unusual situation that can create hundreds of problems in our manufacturing process doesn’t help that. Therefore, the twin must be able to correct the situation it detects. That’s where the advanced solvers or search algorithms come in to find the best combination of parameters for preventing the detected situation.
Evaluation systems must be used again to carry out this search for solutions. Search algorithms for solutions have to work with all possible combinations that exist and evaluate them, taking into account the possible states that the plant might reach when that solution is applied. The calculations for these possibilities are very weighty, and thanks to current information technologies, they can be accelerated, enabling finding the most accurate solution in a short time.
Once the system has been able to determine which of the solutions is best-suited to solving the problem, it is time to generate the appropriate messages that must be sent to every part of the production line. Some of those messages may be sent directly to the machinery, and yet others will have to be handled by the operators to carry out corrective operations. Feedback and the way it is done will depend on the degree of automation in the production process. But this must be done because, without the corrective operation, the non-productive process cannot be rescued.
In short, this final task is what ends up calculating the final solution and sends out the necessary feedback to machines, operators, management, etc. with the ultimate goal of avoiding problems and, like a doctor, helping maintain a healthy production process.
There are no comments yet